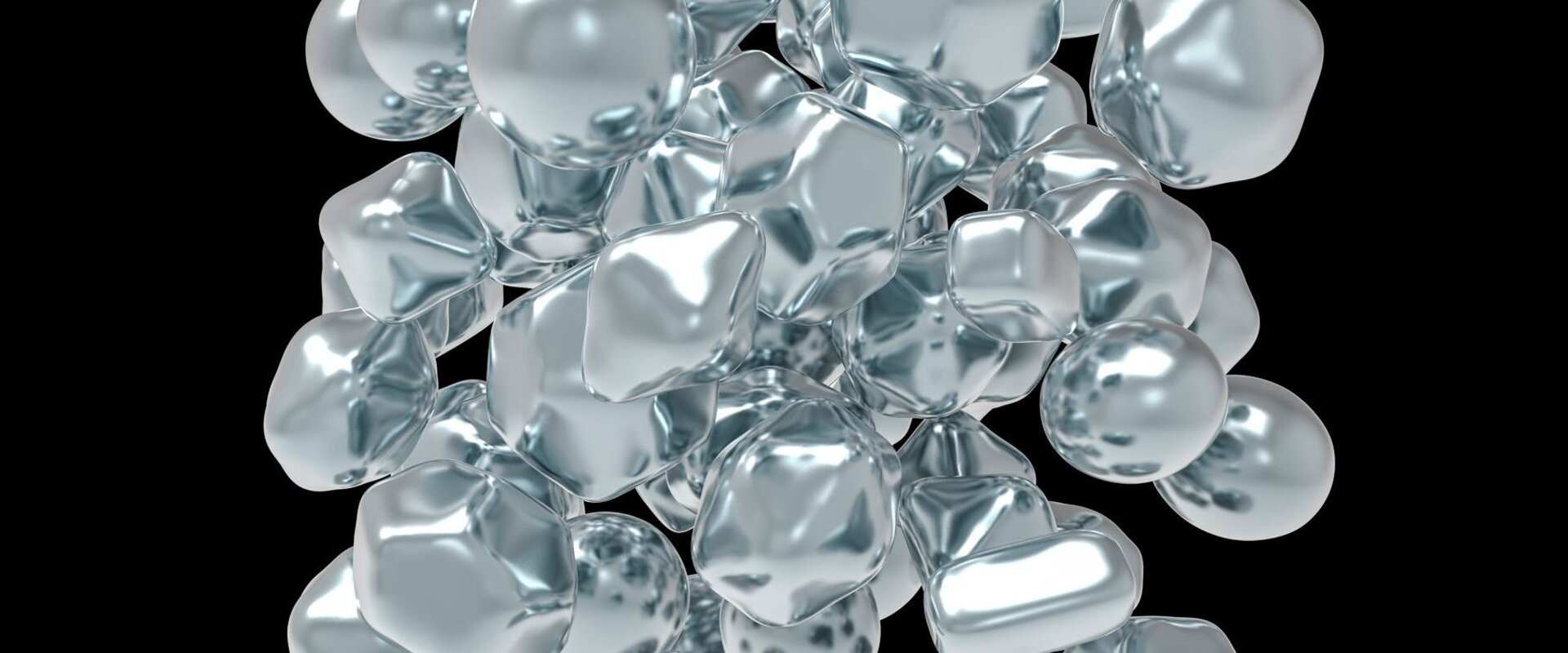
A new deep-learning AI tool for analysing images of complex nanoparticles
Challenge
Nanoparticles (NPs), due to their small size (1-100 nm), have enhanced activity compared to the same material of larger sizes and are finding uses in a range of products and processes, including cosmetics, sunscreens and water treatment. It is estimated that the global market for NPs is €20 billion – with over 300 000 European citizens directly employed by the nanomaterial sector. In addition to the 11 million tonnes produced by the nanotechnology sector, human activities, such as dust from mining, construction and demolition, have greatly increased the number of NPs in the environment. As some NPs possess toxicity, the accurate identification of the types present is essential.
The international standards ISO 21363 and ISO 19749 recommend the use of electron microscopy (EM) which generates images of NPs, allowing identification based on size. However, as some particles may be present as agglomerates and/or aggregates, or have complex shapes, automatic image analysis is problematic. Manual assessment is time consuming and can have day-to-day variability due to small subjective choices between analysts.
An improved method was needed to provide the consistency required both by the nanomaterial industry and environmental and health protection agencies.
Solution
During the nPSize project, POLLEN Metrology applied a software tool they were developing for analysing electron microscopy images of nanostructures and NPs. The early version of the tool required manual identification of NPs upon which the software tool automatically superimposed measurements, such as area and dimensions, including specific diameters and angles. As the project progressed the company incorporated an artificial intelligence (AI) machine learning function, enabling the software to ‘ingest’ large quantities of data to detect recurring patterns, and the system was trained on over 1000 images with more than 15000 annotations from the project partners’ laboratories.
Towards the end of the project in 2021 a ‘deep learning’ process was incorporated and used to analyse more than 15 classes of NPs. This demonstrated the software’s ability to overcome problems associated with edge-effects, agglomerates/ aggregates and non-homogenous samples and accurately identify 1000s of NP images in a few seconds in an automatic, non-subjective way.
Impact
POLLEN Metrology are specialists in providing holistic, AI-driven process and control software for industries and companies aiming to accelerate the time to market for their products. The company’s work in the nPSize project gave them access to 1000s of NP images allowing them to validate the performance of their software, both theoretically and in practice with real data.
The deep-learning function added gives the software the ability to progressively integrate increasingly complex data representations and to ‘transfer learning’ to suit the needs of POLLEN Metrology’s customers. This is now incorporated into the company’s ‘Smart3 software suite’, which not only functions at the nanoscale – but can be scaled up for defect detection for quality control material applications such as silicon wafers or chips for semiconductors, microneedles, electronic displays or any material with a high degree of complexity.
POLLEN Metrology has adapted this technology to compute data other than images, to monitor and model complex processes, and to enrich them over time. It is now being applied to polymer manufacturing processes.
The development of AI analysis tools, like the Smart3 software suite, will not only speed up the identification of potentially harmful nanoparticles – but provide the consistency in data required for a wide range of European industries.
- Category
- EMPIR,
- Standardisation,